Traditional NOC KPIs and Future Cognitive Dark NOC
- Marketing Office
- May 12
- 5 min read
Updated: 6 days ago
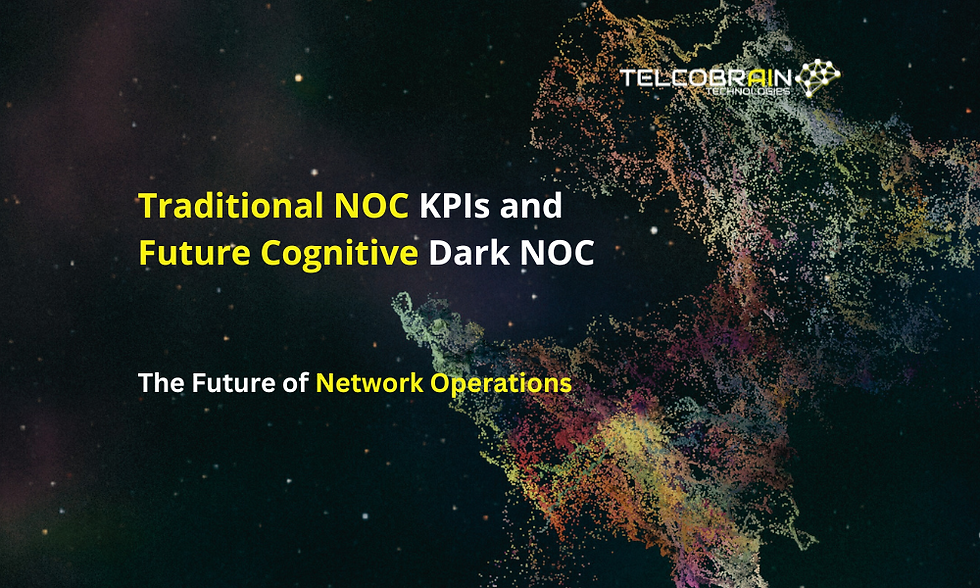
At TelcoBrain, we believe the future of network operations lies in moving beyond manual firefighting or static automation toward a truly intelligent, self‑driving networks. Today’s enterprises and service providers face a growing complexity—from converged IT/OT infrastructures in factories to dynamic 5G, edge‑cloud, and emerging edge-AI services in telecom. To stay ahead, NOCs must evolve, as of today there are two distinct stages: Traditional NOC, Rule‑Based Automation - some may call it Dark NOC. at TelcoBrain we envision a step ahead which is Cognitive Dark NOC, the pinnacle—Agentic AI & Machine Reasoning Autonomy. Each stage not only changes who—or what—runs the network, but also redefines which performance metrics matter most. By embracing the Agentic AI paradigm, TelcoBrain customers unlock a new class of forward‑looking KPIs that shift the focus from “how fast did we fix it?” to “how well do we foresee, prevent, and autonomously resolve issues?”
A Captivating Start: Why NOC Evolution Matters
Imagine a world where network outages are predicted hours—or even days—ahead of customer impact, and corrective actions execute themselves with surgical precision. Manufacturing lines never halt; 5G slices never miss their SLAs; critical cloud services remain uninterrupted. This isn’t sci‑fi—it’s the promise of Agentic AI & Machine Reasoning Autonomy. For too long, NOCs have been trapped in reactive cycles: alerts sound, engineers scramble, tickets close, and then leadership wonders why outages still recur. Static automation brought welcome efficiency gains, but rule sets can’t adapt to novel failures or shifting traffic patterns. Only by empowering AI agents with real‑time reasoning, continuous learning, and self‑healing can organizations transcend firefighting mode and achieve “lights‑out” reliability.
Why KPI Innovation Is Critical
At each evolution stage, the very nature of what you measure—and why—must change. Traditional KPIs like Mean Time to Detect (MTTD) and Mean Time to Resolve (MTTR) tell you how fast humans reacted. Rule‑based automation adds metrics around script success and basic automation ratios. But in an Agentic AI world, the game is about foresight and autonomous resolution: How quickly can the system generate insights? How soon can it act? How reliably does it prevent customer impact? By redefining KPIs, TelcoBrain helps you align network performance with true business outcomes—minimizing revenue at risk, maximizing service availability, and freeing your experts to innovate rather than triage.
NOC Generations: From Human‑Centric to Agentic AI
1. Traditional NOC
Human‑Centric Operations
Who: Engineers and analysts monitor dashboards, parse alerts, and manually execute remediation procedures .
How: Relies on documented processes, ticketing workflows, and human judgment.
Limitations: Scalability bottlenecks, inconsistent response times, and reactive posture.
2. Dark NOC—Rule‑Based with AI/ML Driven Automation
Semi‑Autonomous Operations
Who: Automation engines execute pre‑defined playbooks; humans intervene for novel cases.
How: Static thresholds and rule sets trigger alarms, basic root‑cause steps, and scripted fixes.
Limitations: Handles routine events well but struggles with unforeseen anomalies and dynamic traffic patterns .
3. Cognitive Dark NOC—Agentic AI & Machine Reasoning Autonomy
Fully‑Autonomous, Cognitive Operations
Who: AI agents endowed with reasoning, planning, and self‑healing capabilities operate with minimal human oversight .
How: Continuous learning models detect multivariate anomalies, predict degradations, and close the loop with adaptive remediation strategies .
Advantages: Proactive foresight, instant actions, and resilience in the face of novel failure modes.
KPI Evolution Across NOC Generations
KPI | Traditional NOC | Dark NOC | Cognitive Dark NOC |
MTTD | Time from issue to human acknowledgment via dashboards/tickets | Time from threshold breach to automated alarm (rule trigger) | Superseded by MTTI; ML models detect anomalies in seconds |
MTTR | Time from detection to manual remediation completion | Time from alarm to execution of pre‑defined remediation script | Superseded by MTTA; AI‑orchestrated remediation completes in sub‑minute cycles |
Automation Rate | ~0–10% (simple scripts only) | ~20–40% incidents handled by rule‑based playbooks | 70–90% incidents auto‑remediated by learning agents, improving via reinforcement learning |
MTTI | n/a | n/a | Time from data ingestion to AI‑driven insight—often <10 s |
MTTA | n/a | n/a | Time from insight to action (automated or human‑approved)—typically <60 s |
Proactive Detection Rate | 0% (reactive only) | ~30% via static forecasts (e.g. capacity thresholds) | 70–90% of degradations predicted pre‑SLA impact using ML forecasting |
Self‑Healing Success Rate | 0% (no self‑healing) | ~50% rule‑based fixes succeed without rollback | ~94% AI‑initiated fixes restore KPIs without rollback |
Predictive Accuracy Score | n/a | Informal, no statistical measure | Precision/recall of incident forecasts—routinely >90% |
Anomaly Lead Time | 0 (detects at or after SLA breach) | Minutes–hours for threshold rules | Hours–days of early warning via multivariate anomaly detection |
Network Health Score | Device‑level metrics viewed separately | Per‑domain aggregates via rules | Unified 0–100 health index across IP, wireless, cloud |
AI Model Drift Rate | n/a | n/a | % change in model performance; high drift triggers retraining alerts |
Customer Impact Score | SLA compliance %, CSAT surveys | SLA breaches prevented via rules; manual CSAT | Weighted revenue‑at‑risk × severity × duration—optimized by AI prioritization |
KPI Glossary
MTTD (Mean Time to Detect) Time from issue occurrence to detection.
MTTR (Mean Time to Resolve) Time from detection to resolution of the issue. Automation Rate Percentage of incidents automatically handled without human intervention.
MTTI (Mean Time to Insight) Time from data ingestion to AI-driven insight.
MTTA (Mean Time to Act) Time from insight to execution of corrective action.
Proactive Detection Rate Percentage of issues detected before they impact performance or SLAs.
Self-Healing Success Rate Percentage of automated, AI-driven fixes that successfully resolve issues without rollback.
Predictive Accuracy Score Precision and recall of incident forecasts, measuring the accuracy of predictions.
Anomaly Lead Time Time between early detection of anomalies and when the issue would normally breach SLA.
Network Health Score Unified score representing the overall health of the network, across all layers and domains.
AI Model Drift Rate Measure of AI model performance degradation over time, prompting retraining when necessary.
Customer Impact Score A composite score linking technical KPIs to customer experience and potential revenue impact.
Why These KPI Shifts Unlock New Value
Industrial Enterprises
OT/IT Convergence
MTTI/MTTA reduce detection‑to‑action from hours to seconds, averting costly production halts in SCADA/IIoT environments .
Proactive Maintenance
Anomaly Lead Time transforms maintenance from reactive firefighting into scheduled interventions, boosting Overall Equipment Effectiveness (OEE).
Telecom Operators
5G/6G SLA Assurance
Proactive Detection Rate and Predictive Accuracy ensure slice‑level SLAs are met, reducing churn in competitive markets
OpEx Efficiency
Self‑Healing Success and high Automation Rate cut NOC staffing needs by up to 60%, while improving availability .
Scalability & Resilience
Monitoring AI Model Drift Rate keeps ML models aligned with evolving traffic patterns (e.g. OTT surges), preserving reliability at scale
Final thoughtFrom Firefighting to Foresight
The Time to Act is Now! From manual dashboards to rule-based scripts to self-driving networks, the evolution of the NOC is a story of relentless transformation. The Cognitive Dark NOC is not just the next step—it’s the final leap, where foresight replaces alerts, autonomous agents replace reactive teams, and network operations shift from being a bottleneck to becoming a strategic accelerator.
TelcoBrain's Agentic AI framework based on STAR Loop redefines what’s possible by delivering predictive intelligence, adaptive resilience, and real-time self-healing at scale. With new KPIs like MTTI, MTTA, and Predictive Accuracy Score, our customers gain insight into not just what happened—but what will happen, and how to prevent it. Now is the time to act.
The Digital Infrastructure of tomorrow will not be operated by humans alone—they will be guided by intelligent agents capable of learning, reasoning, and acting autonomously. Those who move first will set the new performance benchmarks. Those who wait will fall behind.
Comments